PreMode
Predicting mode-of-action of missense variants by deep graph representation learning of protein sequence and structural context
This is my second and main PhD project in Dr. Yufeng Shen’s lab. It is the upgraded version of RESCVE.
Accurate prediction of the functional impact of missense variants is important for disease gene discovery, clinical genetic diagnostics, therapeutic strategies, and protein engineering. Previous efforts have focused on predicting a binary pathogenicity classification, but the functional impact of missense variants is multi-dimensional. Pathogenic missense variants in the same gene may act through different modes of action (i.e., gain/loss-of-function) by affecting different aspects of protein function. They may result in distinct clinical conditions that require different treatments. We developed a new method, PreMode, to perform gene-specific mode-of-action predictions. PreMode models effects of coding sequence variants using SE(3)-equivariant graph neural networks on protein sequences and structures. Using the largest-to-date set of missense variants with known modes of action, we showed that PreMode reached state-of-the-art performance in multiple types of mode-of-action predictions by efficient transfer-learning. Additionally, PreMode’s prediction of G/LoF variants in a kinase is validated with inactive-active conformation transition energy changes. Finally, we show that PreMode enables efficient study design of deep mutational scans and optimization in protein engineering.
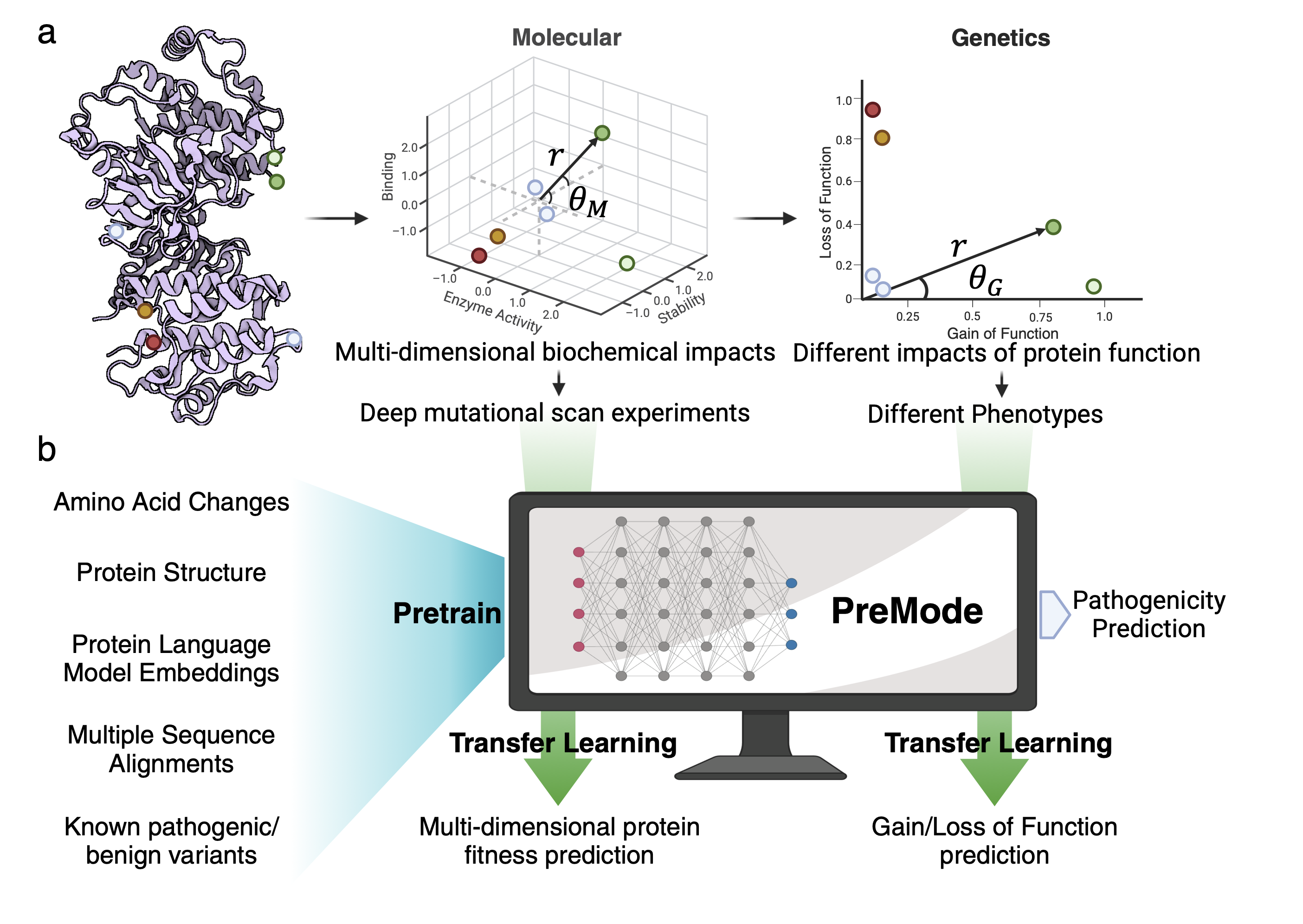
For details, please check our manuscript and GitHub repository.