RESCVE
A deep learning model to represent the modes of action of missense variants.
Accurate prediction of functional impact for missense variants is fundamental for genetic analysis and clinical applications. Current methods focused on generating an overall pathogenicity prediction score while overlooking the fact that variant effect should be multi-dimensional via different modes of action, such as gain or loss of function, and loss of folding stability or enzymatic activity. Recent breakthrough of high-capacity language models enabled ab initio prediction of protein structures as well as self-supervised representation learning of protein sequence and functions. Here we present RESCVE, a method to learn universal representation of sequence variation from protein context. We demonstrated the utility of the method predicting a range of modes of action for missense variants through transfer learning.
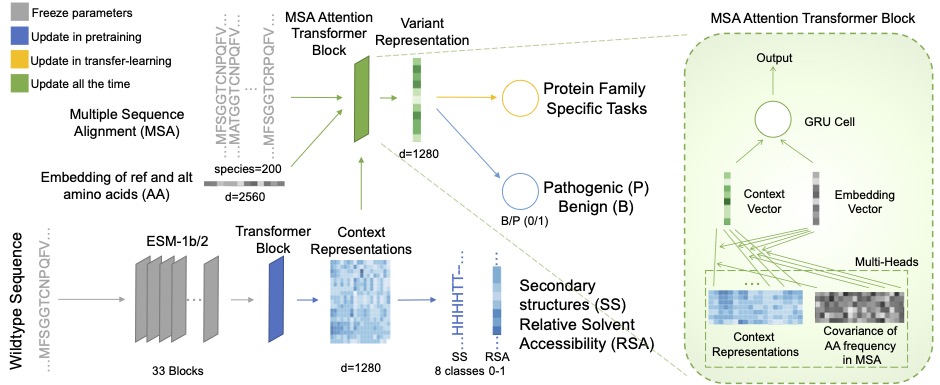
For details, please check our manuscript and GitHub repository.